MGM-AE: Self-Supervised Learning on 3D Shape Using Mesh Graph Masked Autoencoders
Zhangsihao Yang, Kaize Ding, Huan Liu, Yalin Wang
The challenges of applying self-supervised learning to3D mesh data include difficulties in explicitly modeling andleveraging geometric topology information and designingappropriate pretext tasks and augmentation methods for irregularmesh topology. In this paper, we propose a novelapproach for pre-training models on large-scale, unlabeleddatasets using graph masking on a mesh graph composedof faces. Our method, Mesh Graph Masked Autoencoders(MGM-AE), utilizes masked autoencoding to pre-train themodel and extract important features from the data. Ourpre-trained model outperforms prior state-of-the-art meshencoders in shape classification and segmentation benchmarks,achieving 90.8% accuracy on ModelNet40 and 78.5mIoU on ShapeNet. The best performance is obtained whenthe model is trained and evaluated under different maskingratios. Our approach demonstrates effectiveness in pretrainingmodels on large-scale, unlabeled datasets and itspotential for improving performance on downstream tasks.
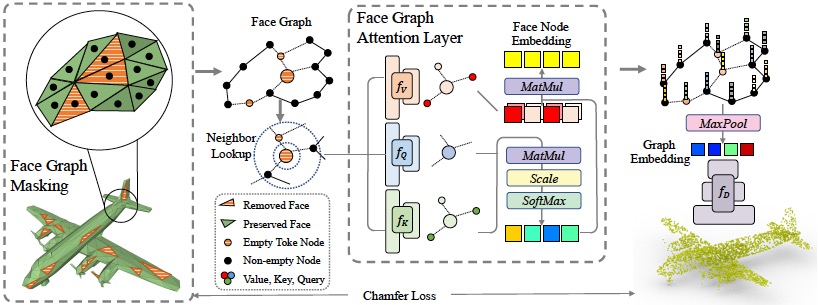
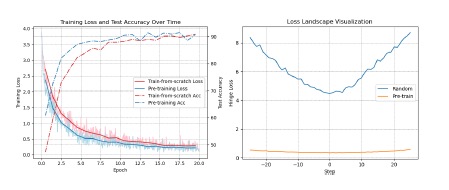
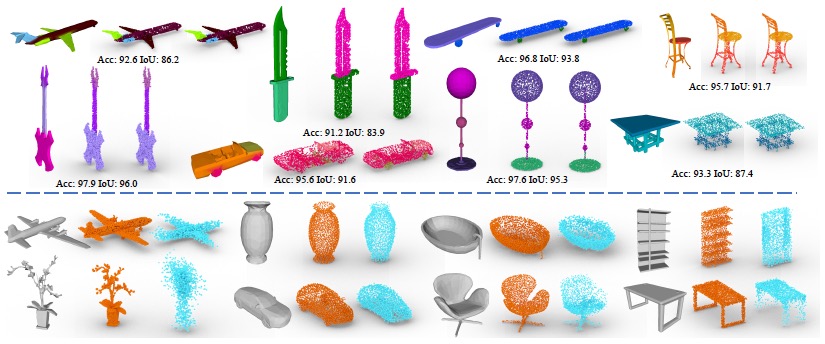