Integrating Structural MRI with Blood-Based Biomarkers for Improved Brain Pathology Detection
Chen Y, Su Y, Wu J, Chen K, Atri A, Caselli RJ, Reiman EM, Wang Y, for the Alzheimer’s Disease Neuroimaging Initiative
Background: Alzheimer’s disease (AD) is the most prevalent form of age-related dementia, impacting 6.2 million people aged 65 or older as indicated by CDC data. The presence of amyloid plaques and neurofibrillary tangles are among the earliest signs of Alzheimer’s disease (AD), preceding any cognitive impairment and brain structure alterations. Positron emission tomography (PET) enables direct measurement of brain Aβ and tau pathology, but its limited accessibility and cost have prompted the exploration of alternative diagnosis methods utilizing more widely available magnetic resonance imaging (MRI) or blood-based biomarkers (BBBs).
Methods: We applied our previously developed PASCS-MP framework to extract hippocampal multivariate morphometry statistics (MMS) features from MR images. Together with blood-based biomarker plasma Aβ42/40, we trained a random forest classifier to perform a binary classification of patients’ brain amyloid positivity. The model performance was assessed based on prediction accuracy, precision, recall rate, F1 score and AUC score.
Results: We evaluated the performance of our integrated model on two distinct datasets, one from Alzheimer’s Disease Neuroimaging Initiative (ADNI) database and the other from Banner Alzheimer’s Institute (BAI). In comparison to the two baseline models that solely utilized either MMS features or plasma Aβ42/40 as predictors, our integrated model demonstrated superior performance. The combined model achieved prediction accuracies of 0.85 ± 0.01 on ADNI dataset and 0.90 ± 0.01 on BAI dataset, respectively, which are significantly higher than the baseline models.
Conclusion: In this study, we developed an integrated model that combines featured extracted from MR images and blood-based biomarkers to predict brain amyloid positivity of Alzheimer’s disease patients. Our integrated model outperformed the baseline models that relied solely on MRI data or blood-based biomarkers. This approach offers a promising avenue for leveraging more accessible and cost-effective techniques in AD diagnosis, such as MRI and blood-based biomarkers, as alternatives to expensive and less available diagnosis methods like Tau-PET. This study was among the first set of methods to integrate MRI and blood-based biomarkers for AD diagnosis.
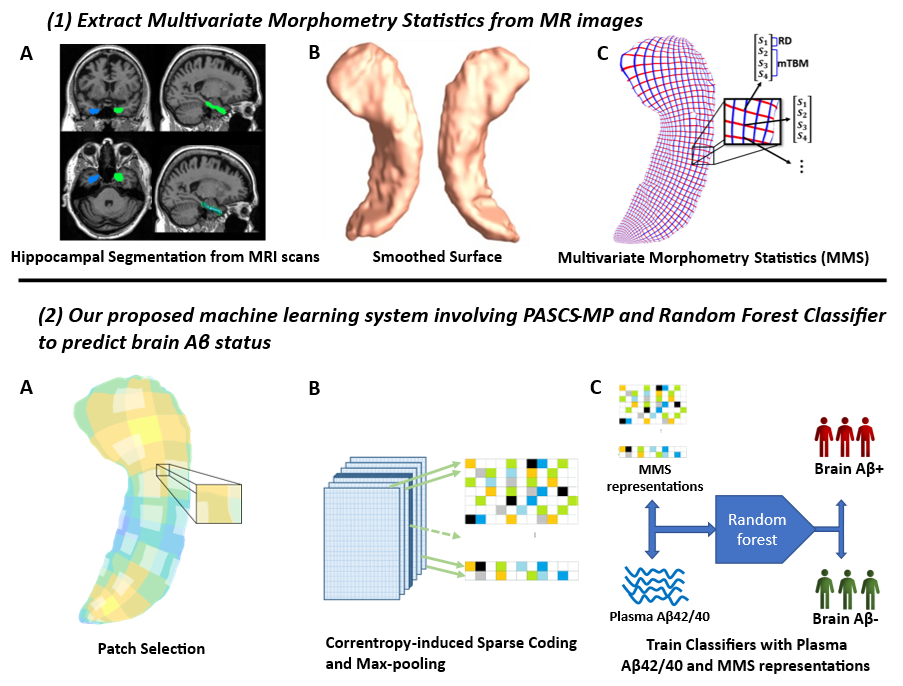
The new website is finally online!
Hope it will privide a good demostration of our work.