TimeMIL: Advancing Multivariate Time Series Classification via a Time-aware Multiple Instance Learning
Chen X*, Qiu P*, Zhu W*, Li H, Wang H, Sotiras A, Wang Y, Razi A
Accepted by the 41st International Conference on Machine Learning (ICML), (* indicates equal contributions)
Abstract: Deep neural networks, including transformers and convolutional networks, have significantly enhanced multivariate time series classification. However, they often rely on supervised learning, which may not fully account for the sparsity and locality of time series patterns, such as ECG anomalies. To address this, we propose TimeMIL, a novel multiple-instance learning framework that enhances localization of key patterns and captures time dependencies in time series. TimeMIL uses time-aware MIL pooling and a specialized learnable positional token within a transformer. This method surpasses existing methods, demonstrating the effectiveness of weakly supervised learning in handling time series classification tasks.



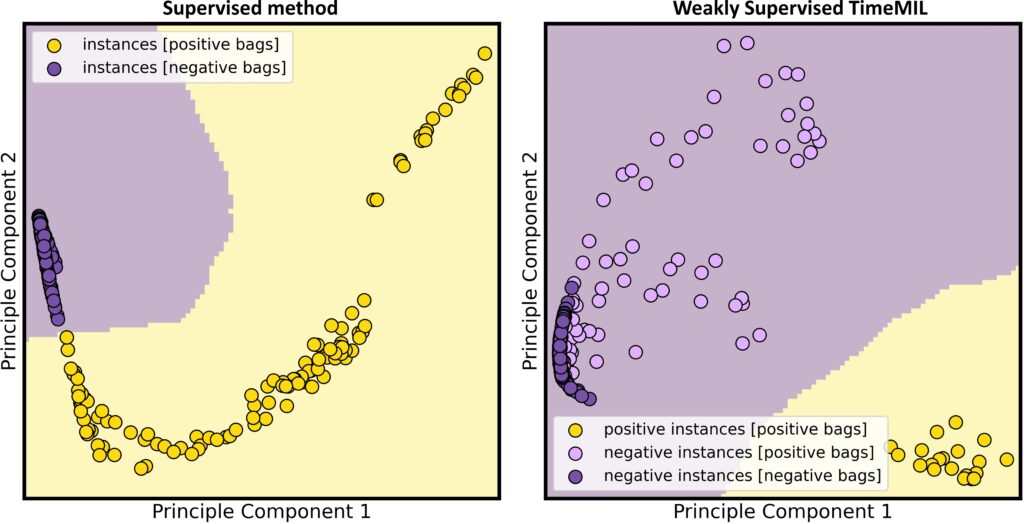
